I. Introduction: Outdated Assumptions in the Age of AI
The unauthorized use of copyrighted works to train generative AI models is the greatest threat to the continued value and viability of human creative labor. Ever.
Our instinct, in both law and society, has long been the assumption that “publicly available means fair game.” If it’s visible—on a street corner, in a bookstore, or on a website—it’s treated as open for unrestricted use. That assumption once shaped early Fourth Amendment law, and it currently underlies the justification for large-scale AI scraping of creative works without permission and the rendering of original creators to ghostwriters to their own cultural legacy.
But technological leaps can change not just the extent of the harm but its very nature. The Supreme Court recognized this in Katz v. United States,1 when the Court pulled back from the rigid idea that what’s in public is categorically unprotected from government surveillance. This reflected an awareness that the use of technology can intrude on reasonable expectations of privacy—even in public or semi-public spaces.
Copyright law now faces a moment of the same magnitude.2 The idea that the availability of creative works amounts to a right to train AI to replace the very creators of those works—without express authorization to do so and without limitation—requires careful examination and, for some, soul-searching.3
II. The Core Defense: Fair Use, or the Fiction of Learning
Virtually all legal defenses by LLM providers to their unauthorized use of copyrighted works for training originate from their invocation of the fair use doctrine.
We will reserve our deep-dive into the fair use defense for next time, but one thread of the argument goes like this: just as humans learn by reading and synthesizing knowledge, so too do AI models “learn” from the data they consume. If human learning isn’t infringement, the reasoning goes, neither should machine learning be. LLM developers contend that while a particular output may be informed by an original copyrighted work, it is also shaped by countless other sources, and any resemblance to one specific work is incidental—not the result of copying in the legal sense.
Whether courts will accept this analogy, however, remains very much in dispute—and depends in part on how they interpret the scale, precision, and retention power that distinguish LLMs from human minds.
III. From Surveillance to Substitution: Technology Changes Doctrine
Legal doctrine often lags behind technological disruption. The law’s assumptions—about what’s visible, what’s public, and what’s fair—can collapse when scale and precision transform the nature of harm. That’s exactly what happened with the Fourth Amendment in the era of government surveillance, and it’s what’s now unfolding in copyright law as AI systems convert public access into the great replacement—of human authorship, not humanity.
A. The evolution of Fourth Amendment doctrine with modern technological surveillance
For much of the 20th century, Fourth Amendment jurisprudence followed a rigid logic: what was exposed to public view was unprotected. If someone left their curtains open or spoke in a public place, they had no “reasonable expectation of privacy.” But as surveillance capabilities advanced, that premise began to break down.
The turning point came in Katz, where the Supreme Court held that a person using a public phone booth could still retain a reasonable expectation of privacy—not because the setting was private in the physical sense, but because the user’s intent to keep the conversation private deserved constitutional recognition.4 What mattered was not merely visibility, but how technology amplified the power to observe and exploit.
Subsequent cases—addressing GPS trackers, cell-site location data, and dragnet surveillance—have reaffirmed that the Fourth Amendment must evolve when new technologies allow public behavior to be captured, aggregated, and weaponized in ways never before possible.5
[cont’d ↗]
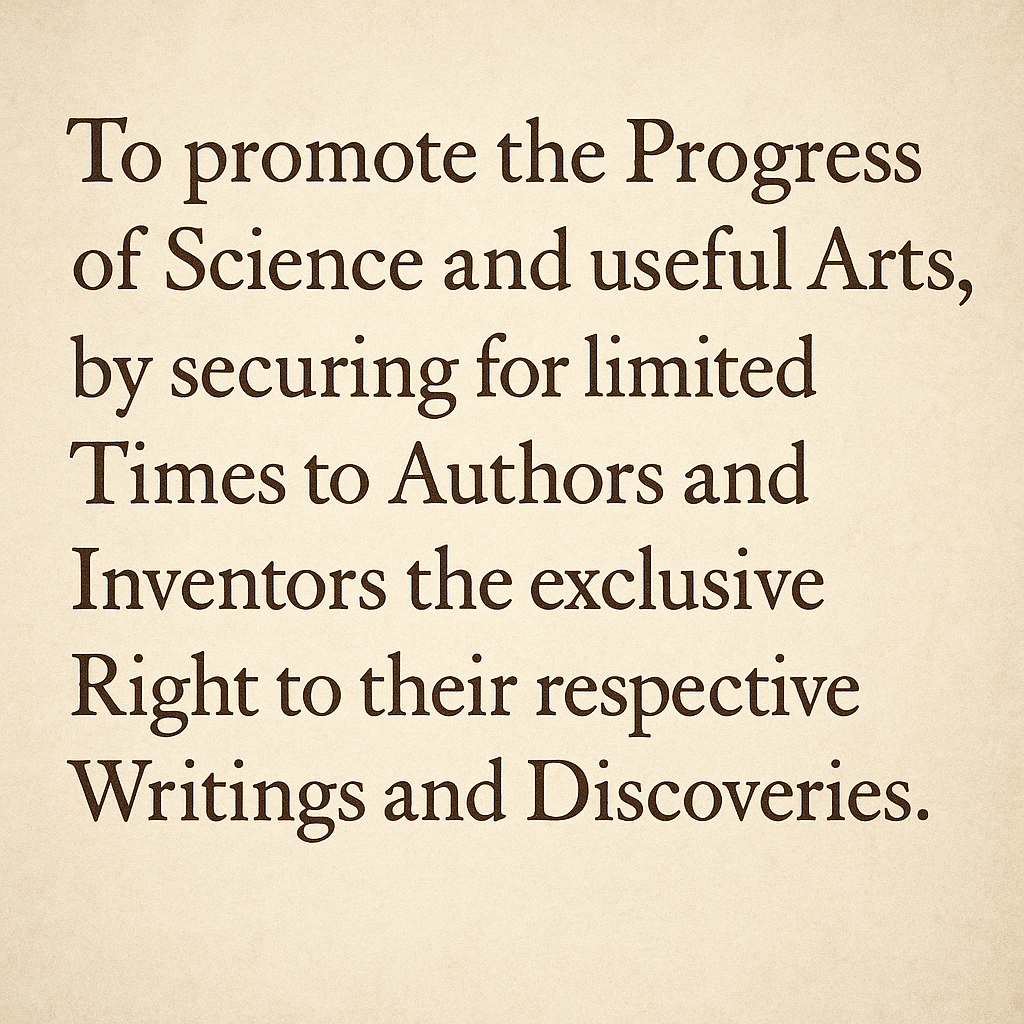
U.S. Const. Art. I, Sec. 8, Cl. 8. But what do we do when the “progress of science” and the “exclusive right” of authors and other creators to their creations are in conflict? See Part 2 of this article, next week
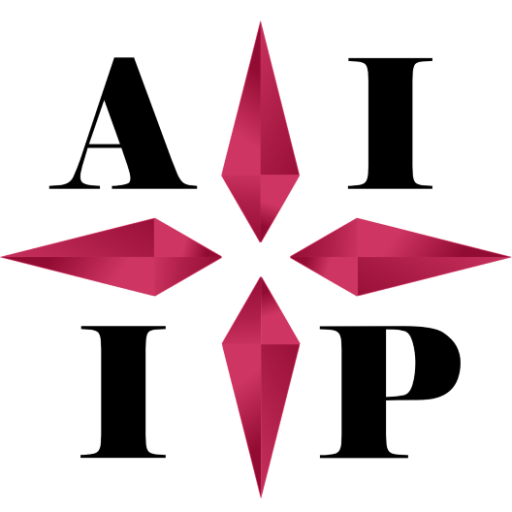

Self-schedule a free 20-min. video or phone consult with Jim W. Ko of Ko IP and AI Law PLLC here.
B. Copyright and AI: Why AI training is not analogous to human learning
That same logic now confronts copyright law. Courts, creators, and companies are grappling with whether the public availability of creative works online gives AI systems license to ingest, retain, and reproduce their expressive features—without consent or compensation.
It’s true that copyright regulates private conduct, not state actors. But the doctrinal echo is clear: both surveillance and AI training exploit a core assumption that public availability implies lawful use. In reality, scale and automation have changed the stakes. A human browsing a few blog posts is categorically different from an LLM trained on millions of blog posts to mimic their structure and style. And just as courts came to see that a thermal imaging scan or GPS tracker was not “just observation,” courts must now recognize that LLM training is not “just reading.”
Just because works are publicly posted, it does not mean they are in the public domain. Even without a copyright notice, original works fixed in a tangible medium are presumptively protected under U.S. law.6 The Copyright Act of 1976 eliminated formalities like mandatory notice or registration as a condition of protection.7 Yet the myth persists that if a work is online—and unmarked—it’s free for any use. That misunderstanding is now weaponized at industrial scale.
1. Machines don’t learn like humans
The fair use defense often hinges on the analogy between machine and human learning. But legally and functionally, the analogy breaks down.
Humans interpret. Machines retain. A person reading ten articles may be influenced by them, but they forget, misremember, and synthesize imperfectly. An LLM ingests entire corpora, transforms expressive language into vectorized representations, and reuses those patterns with near-perfect recall.
The concern isn’t that any given AI-generated output is a direct or substantially similar copy of one original work. In most cases, it isn’t. The deeper issue lies in the front-end: LLMs are trained through the wholesale ingestion of protected works—many in their entirety—without authorization.
The real concern is that AI models now generate content that fulfills the same purpose, echoes the same tone, and competes in the same market—replacing the original work and potentially displacing the author who created it.
2. The quantitative and qualitative gap we cannot ignore
What makes LLM training an entirely different animal isn’t just how it functions—it’s how much it consumes and how broadly it can deploy.
- Volume: LLMs are trained on hundreds of thousands, even millions, of copyrighted works—often in their entirety.
- Speed: A human might read a few books in a week. An AI model processes terabytes of content in hours.
- Scale of output: A person might publish a few articles a year. A deployed LLM can generate thousands of derivative works daily, many competing directly with human-authored content.
These differences are not incidental—they are foundational. Just as courts eventually recognized that digital surveillance changed the nature of privacy violations, they must now grapple with how generative AI changes the nature of unauthorized use.
V. Conclusion: A Law Written for the Past Must Answer to the Present
Technology oft outpaces the law. That’s not new. And it’s rarely permanent.
But step back far enough, and you’ll often find that the law contains latent tools—failsafes that can be activated through principled interpretation. Whether rooted in statutory text, common law traditions, or constitutional structure, those tools can and should be brought to bear when disruption reshapes the ground beneath a doctrine. That is certainly the case with the fair use doctrine, which—true to its name—was always intended to be fair, not a loophole so broad it swallows the rule.
Copyright law is at such a crossroads now. And it is up to our courts, our legislators, and—most importantly—our people to determine how to balance the rights of:
- Individual creators, whose exclusive right is constitutionally protected “to promote the Progress of Science and the useful Arts”; and
- LLM providers, who are competing for global AI dominance in a race that will shape the future of information, authorship, and power …
… for the enduring benefit of society.
© 2025 Ko IP & AI Law PLLC
In Part 2, we’ll go far more in depth on the fair use doctrine—the core defense raised by LLM providers in pending AI/copyright litigation such as The New York Times Co. v. Microsoft Corp. and Andersen v. Stability AI. We’ll examine whether the fair use test is capable of meaningfully addressing AI’s speed, scale, and substitutional power.
- Katz v. United States, 389 U.S. 347, 351–53 (1967) (holding that the Fourth Amendment protects people, not places, and establishing that individuals may retain a reasonable expectation of privacy even in public settings, including from warrantless governmental wiretapping of a public telephone booth). ↩︎
- As discussed in my recent blog article, defining the proper boundaries of rights—whether the Fourth Amendment’s protection against government intrusion or copyright’s shield against public and private encroachment—requires value judgments that AI can simulate but not make. What Getting Fired Taught Me About Due Process—Why AI Can’t Deliver Justice…, available here. There is no empirically “correct” answer. Balancing competing legitimate interests is a human task, guided by law, public discourse, and ultimately legislative action. Only humans can weight the coefficients for AI models to reflect competing values. If AI begins to do so on its own, without explicit human direction, then human judgment is no longer merely displaced; it is abdicated. ↩︎
- The U.S. Copyright Office issued its long awaited report on Generative AI Training last week concluding the same. See U.S. Copyright Office, Copyright and Artificial Intelligence: Part 3 – Generative AI Training (May 2025) (pre-publication version), stating that “making commercial use of vast troves of copyrighted works to produce expressive content that competes with them in existing markets, especially where this is accomplished through illegal access, goes beyond established fair use boundaries.” Available here.
Shortly after the release of this report, the Trump administration dismissed Shira Perlmutter, Director of the U.S. Copyright Office, without providing an official rationale. ↩︎ - See supra note 1. ↩︎
- For discussion of the case law concerning technological surveillance and its potential applicability in the AI Age, see The Federal Judicial Center, An Introduction to Artificial Intelligence for Federal Judges, Sec. 8 (AI in the Courtroom), Fourth Amendment, at 63-70 (2023), available here. ↩︎
- See 17 U.S.C. § 102(a) (providing copyright protection for “original works of authorship fixed in any tangible medium of expression”); 17 U.S.C. § 401(a) (making use of a copyright notice optional, and clarifying that lack of notice does not invalidate copyright). ↩︎
- Practice tip: you should still mark all your works of authorship with a copyrighted designation (e.g., Copyright or © 2025 Ko IP & AI Law PLLC). While not required, this makes the protected status of your creative works unequivocal, deters misuse (well, at least by some humans), and increases the enforceability of your copyrights. ↩︎
Leave a Reply